In the ever-evolving landscape of understanding our world, the approaches we take to dissect and comprehend complex systems are critical. These systems, whether they are ecosystems, economies, or social structures, present intricate challenges that demand nuanced methodologies. Two prominent approaches stand out: one that leans heavily on data-driven analysis and computational power, and another that embraces a more holistic, participatory, and adaptive perspective. This article delves into these contrasting philosophies, exploring their strengths, weaknesses, and implications for navigating the complexities of the 21st century.
The Allure of Data and Computation: A Quest for Control
The first approach is characterized by its reliance on vast datasets, advanced computational algorithms, and a desire for predictive accuracy and control. This perspective is deeply rooted in the traditions of reductionist science, which seeks to understand phenomena by breaking them down into their constituent parts and analyzing their interactions. In this framework, the assumption is that with enough data and processing power, we can model complex systems with sufficient fidelity to predict their behavior and intervene effectively. Learn about Data-Driven Approaches.
This data-centric approach is often justified by the exponential growth of data availability and computational capabilities. Moore’s Law, which posits that the number of transistors on a microchip doubles approximately every two years, has fueled the rapid advancement of computing power. This has led to the development of sophisticated tools and techniques, such as machine learning and artificial intelligence, which can analyze large datasets and identify patterns that would be impossible for humans to detect.
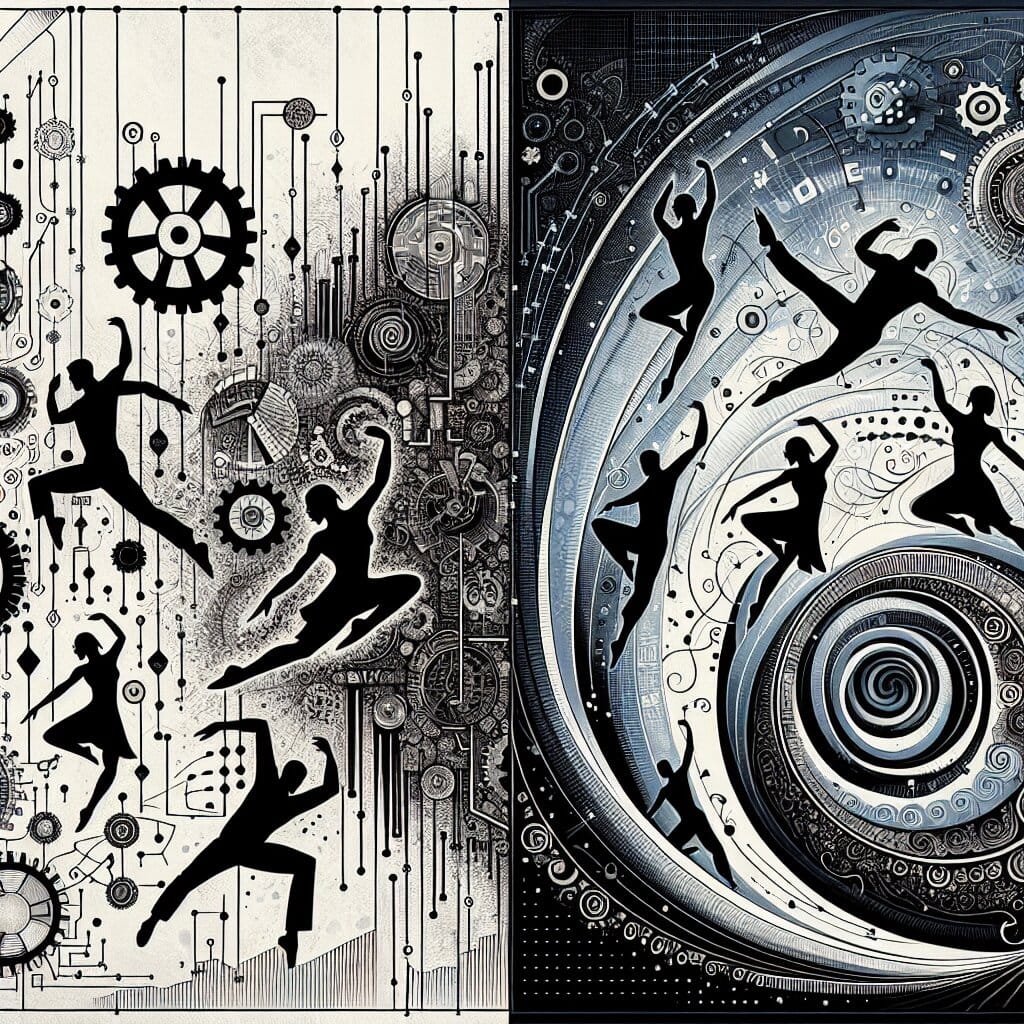
Real-World Applications and Examples
One prominent example of this approach is in the field of climate modeling. Climate scientists use complex computer models to simulate the Earth’s climate system, incorporating data on temperature, precipitation, greenhouse gas concentrations, and other variables. These models are used to project future climate scenarios and inform policy decisions aimed at mitigating climate change.
Another example is in the field of finance, where algorithms are used to analyze market data and make trading decisions. High-frequency trading firms, for instance, rely on sophisticated algorithms to identify and exploit fleeting market inefficiencies, executing trades in milliseconds.
In the realm of public health, data analytics are used to track the spread of infectious diseases and develop targeted interventions. For example, during the COVID-19 pandemic, data on infection rates, hospitalizations, and mortality were used to inform public health measures such as lockdowns, mask mandates, and vaccination campaigns.
Historical Context: The Roots of Reductionism
The data-driven approach to complex systems has its roots in the scientific revolution of the 17th century, which saw the rise of mechanistic philosophy and the development of calculus. Figures such as Isaac Newton and René Descartes championed the idea that the universe could be understood as a machine governed by deterministic laws. This perspective led to the development of powerful tools for analyzing and predicting physical phenomena, but it also fostered a belief in the possibility of complete knowledge and control.
Expert Opinions and Perspectives
Many scientists and engineers embrace the data-driven approach, arguing that it offers the best hope for understanding and managing complex systems. They point to the successes of data analytics in fields such as medicine, engineering, and finance as evidence of its effectiveness.
For example, Pedro Domingos, a professor of computer science at the University of Washington, argues in his book “The Master Algorithm” that machine learning holds the key to unlocking the secrets of intelligence and creating machines that can solve complex problems.
Limitations and Challenges: The Pitfalls of Control
Despite its allure, the data-driven approach to complex systems faces several limitations and challenges. These challenges stem from the inherent characteristics of complex systems, such as nonlinearity, feedback loops, and emergent behavior. Learn about Complex Systems at MIT.
Energy Constraints
Relying on data and computation to control complex systems depends on massive computation, which depends on the very physical elements of electricity, rare metals and other material inputs, and extremely complex supply chains. Computation is a product of high-energy modernity, which has been shown repeatedly to be unsustainable. Don’t forget to see Data Insights on Federal Spending, Workforce, and Public Datasets.
Chaos Theory
Complex systems are often characterized by chaotic behavior, which means that small changes in initial conditions can lead to large and unpredictable outcomes. This phenomenon, known as the “butterfly effect,” makes it difficult to predict the long-term behavior of complex systems, even with the most sophisticated models.
The Logistic Equation
Consider the logistic equation, which is a mathematical relationship used to demonstrate chaos. The equation states that the value at any given time step can be calculated by a simple transformation of the value at the preceding time step. If you know the initial value with complete precision, it’s easy to predict. But if we plug in an estimate of the initial value and we are off by a teeny tiny amount, our prediction can be way off from reality just a few steps down the road. As you extend the time period that you want to predict, the amount of precision you need in measuring the initial condition increases exponentially.
Entropy and Unintended Consequences
The Second Law of Thermodynamics, which describes the tendency for energy to spread out over time from more useful, concentrated bundles to less useful, distributed messes states that in order to reduce the entropy in one area, entropy must be increased elsewhere. As a result of the Second Law, if you attempt to solve a problem like climate change by controlling the complex systems that drive it, you may find yourself playing whack-a-mole as unintended disorderly consequences pop up in connected systems. Perhaps you solved carbon emissions, but crashed the economy and crushed biodiversity.
The Observer Effect
If you seek control of complex systems, you are necessarily assuming a separation between the controller and the thing to be controlled. There are some things you just can’t understand unless you engage with them. Check out “Choosing Your Path in Data Science and Engineering Careers“.
The Dance of Systems: Embracing Complexity and Adaptation
In contrast to the data-driven approach, the “dance of systems” emphasizes the importance of understanding complex systems as interconnected wholes, rather than as collections of isolated parts. This approach recognizes that complex systems are constantly evolving and adapting, and that attempts to control them can often lead to unintended consequences. Check out “Systems Thinking” at Kumu.
Donella Meadows and Systems Thinking
The concept of “dancing with systems” is closely associated with the work of Donella Meadows, a systems thinker and author of the influential book “Thinking in Systems.” Meadows argued that the most effective way to manage complex systems is to understand their underlying dynamics and to work with them, rather than against them.
Key Principles of the “Dance of Systems”
- Embrace uncertainty: Acknowledge that complex systems are inherently unpredictable and that our understanding of them will always be incomplete.
- Focus on feedback loops: Pay attention to the feedback loops that shape the behavior of complex systems and identify opportunities to intervene in ways that promote stability and resilience.
- Promote diversity and redundancy: Encourage diversity and redundancy in complex systems to make them more resilient to shocks and disturbances.
- Foster collaboration and communication: Promote collaboration and communication among stakeholders to ensure that everyone is aware of the system’s dynamics and is working towards common goals.
- Learn from experience: Continuously monitor the behavior of complex systems and learn from our successes and failures.
Learn about Adaptation and Uncertainty.
Real-World Examples of the “Dance of Systems”
- Ecosystem management: Instead of trying to control ecosystems through top-down interventions, ecosystem managers can work with natural processes to promote biodiversity, resilience, and ecosystem services.
- Community development: Instead of imposing solutions from the outside, community developers can work with local residents to identify their needs and priorities and to create solutions that are tailored to their specific context.
- Organizational change: Instead of trying to force change through rigid plans and directives, organizational leaders can create a culture of learning and adaptation that allows organizations to evolve in response to changing circumstances.
You should read about “Land Your Dream Data Science Internship: A Complete Guide!“.
Historical Context: The Rise of Systems Thinking
The “dance of systems” approach has its roots in the systems thinking movement, which emerged in the mid-20th century as a response to the limitations of reductionist science. Systems thinkers such as Ludwig von Bertalanffy and Jay Forrester argued that the world is best understood as a collection of interconnected systems, rather than as a collection of isolated parts. You may be interested in “Amazing Dark Universe Discoveries: UK Science Probes Cosmic Mysteries!“.
Expert Opinions and Perspectives
Many scientists, practitioners, and policymakers are embracing the “dance of systems” approach, arguing that it offers a more effective way to manage complex challenges such as climate change, poverty, and inequality.
For example, Elinor Ostrom, a Nobel Prize-winning economist, demonstrated that communities can effectively manage common-pool resources such as forests and fisheries by establishing clear rules and norms and by fostering collaboration and communication. Check out “Exciting Generative AI Trends You Need to Know!“
Finding Balance: Integrating Data and Dance
The data-driven approach and the “dance of systems” are not mutually exclusive. In fact, the most effective way to navigate complex systems may be to integrate these two approaches, using data and computation to inform our understanding of system dynamics, while also embracing the uncertainty and adaptability that are inherent in complex systems.
By combining the power of data analytics with the wisdom of systems thinking, we can develop more effective strategies for managing complex challenges and creating a more sustainable and equitable world. You may be interested in “Amazing Data Science CEO: Emily Saras Inspires!“
In conclusion, whether we choose to collect huge amounts of data and use it to predict and ultimately control complex systems or to treat complex systems not as objects we can predictably control, but as partners in a great dance, there are positives and negatives to both. By combining the power of data analytics with the wisdom of systems thinking, we can develop more effective strategies for managing complex challenges and creating a more sustainable and equitable world.
Word Count: 2416 “`