Schneider Electric and ETAP have launched a groundbreaking digital twin tool designed to transform the design, management, and optimization of data centers for Artificial Intelligence (AI) workloads, often termed “AI factories.” This collaboration, presented at Nvidia’s GTC event, integrates ETAP’s electrical engineering expertise with Schneider Electric’s comprehensive data center solutions and Nvidia’s Omniverse platform to create a sophisticated virtual environment. The tool offers detailed simulations of mechanical, thermal, networking, and electrical systems, providing unprecedented insights into the performance and efficiency of these critical facilities.
The implications of this development are profound, especially given the increasing demands of AI applications. As AI models become more complex and data-intensive, the infrastructure required to support them must evolve to meet these escalating needs. Traditional data centers often struggle to handle the power, cooling, and connectivity requirements of AI workloads, leading to inefficiencies and potential bottlenecks. The new digital twin tool addresses these challenges by providing a virtual replica of the data center, enabling operators to optimize their infrastructure for AI and improve operational resilience.
The Rise of AI Data Centers and Their Unique Challenges
The term “AI factory” emphasizes the specialized nature of data centers designed to support AI workloads. Unlike traditional data centers, which primarily focus on general-purpose computing, AI factories are optimized for the specific demands of AI training and inference. These workloads are characterized by:
- High Compute Density: AI training requires massive parallel processing capabilities, typically achieved through the use of GPUs (Graphics Processing Units) or specialized AI accelerators. This results in a high concentration of computing power within a relatively small area.
- Extreme Power Consumption: The intensive computations performed by AI hardware consume significant amounts of electrical power. This not only increases operating costs but also poses challenges for power distribution and cooling systems.
- Stringent Cooling Requirements: High power consumption translates directly into increased heat generation. Effective cooling solutions are essential to prevent overheating and maintain optimal performance. This often involves advanced cooling technologies such as liquid cooling or direct-to-chip cooling.
- Low-Latency Networking: AI applications often require high-bandwidth, low-latency communication between servers, storage, and network devices. This necessitates a robust and efficient network infrastructure capable of handling the demands of distributed AI training.
The increasing demand for AI-driven applications in various industries, including healthcare, finance, and transportation, is driving the growth of AI data centers. According to a report by Gartner, the global AI software market is projected to reach $62.5 billion in 2022, an increase of 21.3% from 2021. This growth is expected to further accelerate the demand for AI infrastructure, including specialized data centers.
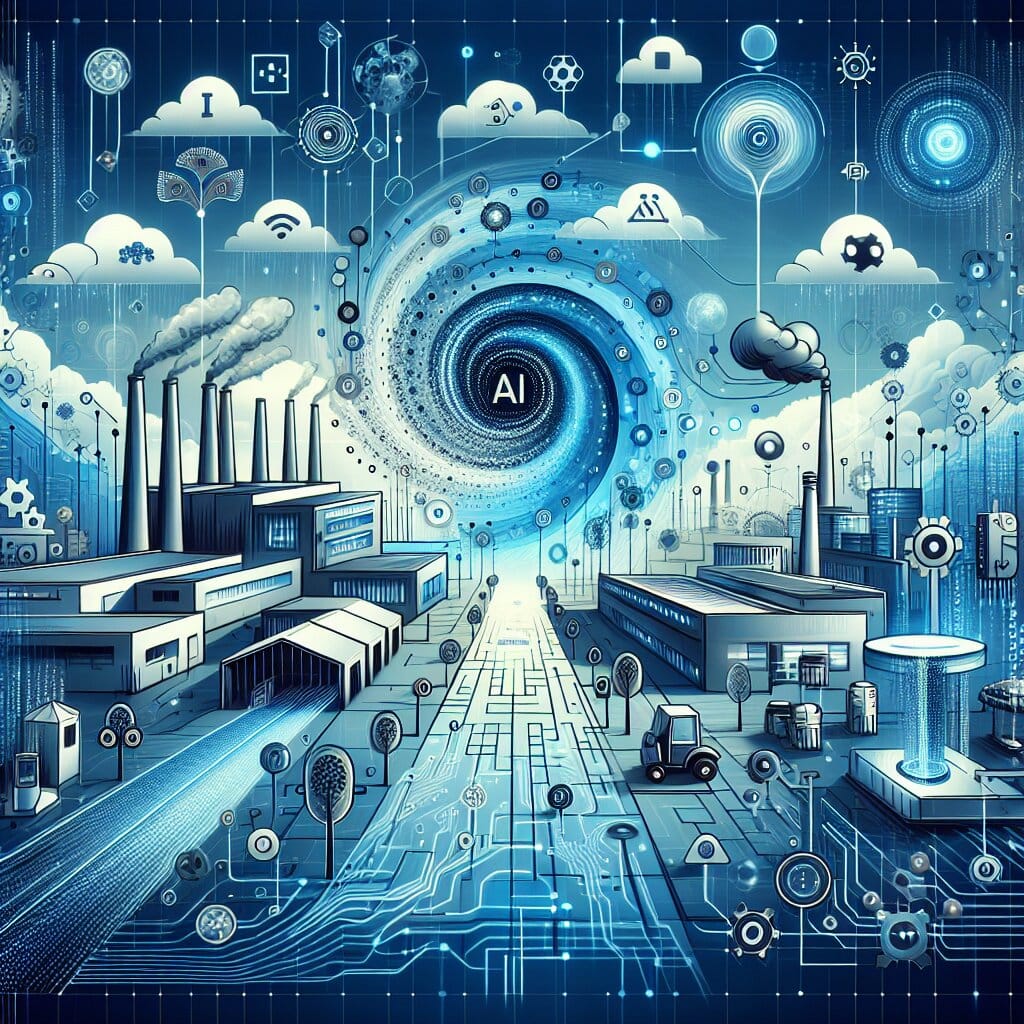
Understanding Digital Twins: A Revolution in Data Center Management
A digital twin is a virtual representation of a physical asset or system, created using real-time data and advanced simulation techniques. In the context of data centers, a digital twin can encompass the entire facility, including its physical infrastructure, IT equipment, and operational processes. The digital twin is continuously updated with data from sensors, meters, and other monitoring devices, providing a dynamic and accurate reflection of the data center’s current state.
The concept of digital twins has been around for decades, initially used in aerospace and manufacturing industries. However, its application to data centers is relatively recent, driven by advancements in computing power, sensor technology, and data analytics. The benefits of using digital twins in data center management are numerous:
- Improved Design and Planning: Digital twins enable data center designers to simulate different configurations and evaluate their performance before construction begins. This helps optimize space utilization, power distribution, and cooling efficiency.
- Enhanced Operational Efficiency: By providing real-time visibility into the data center’s operations, digital twins enable operators to identify and address inefficiencies. This can lead to reduced energy consumption, improved resource utilization, and lower operating costs.
- Predictive Maintenance: Digital twins can be used to predict equipment failures and schedule maintenance proactively. By analyzing historical data and real-time sensor readings, the digital twin can identify patterns that indicate potential problems. This helps prevent downtime and extend the lifespan of critical equipment.
- Optimized Capacity Planning: Digital twins can help data center operators optimize their capacity planning by predicting future resource requirements. By simulating different scenarios, operators can determine when additional capacity will be needed and plan accordingly.
- Faster Incident Response: In the event of an incident, such as a power outage or cooling failure, the digital twin can provide operators with a clear picture of the situation and help them quickly identify the root cause and implement corrective actions.
Historical Context and Evolution of Digital Twins
The origin of the digital twin concept can be traced back to NASA’s Apollo program in the 1960s. The space agency used paired vehicles to mirror the conditions of spacecraft in flight, allowing engineers to simulate scenarios and troubleshoot issues in real-time. This early form of digital twinning helped ensure the safety and success of space missions. The term “digital twin” itself was formally coined by Dr. Michael Grieves at the University of Michigan in 2002. He proposed the digital twin as a conceptual model for product lifecycle management, emphasizing the importance of a virtual representation mirroring a physical entity throughout its lifecycle.
Over the years, digital twins have evolved significantly, driven by advancements in computing power, sensor technology, and data analytics. Early applications were primarily in aerospace and manufacturing, where the high value of assets justified the investment in sophisticated simulation tools. As technology has become more accessible and affordable, digital twins have found applications in a wider range of industries, including healthcare, energy, and urban planning. In healthcare, for example, digital twins are being used to create virtual models of patients, allowing doctors to simulate treatments and predict outcomes. In the energy sector, digital twins are used to optimize the performance of power plants and predict maintenance needs. In urban planning, digital twins are used to model cities, simulate traffic patterns, and optimize infrastructure investments.
Real-World Examples of Digital Twin Applications
Several organizations have successfully implemented digital twins to improve their operations and reduce costs. For example, Siemens has developed a digital twin platform called MindSphere, which is used to optimize the performance of industrial equipment. MindSphere connects to sensors on machines and analyzes the data to identify potential problems and improve efficiency. GE Aviation uses digital twins to monitor the performance of aircraft engines. By analyzing data from sensors on the engines, GE can predict maintenance needs and prevent downtime. The city of Singapore has created a digital twin of the entire city, which is used to simulate traffic patterns, optimize infrastructure investments, and improve emergency response. The digital twin incorporates data from a variety of sources, including sensors, cameras, and government databases.
The Schneider Electric-ETAP Digital Twin Solution: Features and Functionality
The digital twin tool developed by Schneider Electric and ETAP builds upon the established principles of digital twins, incorporating advanced features specifically tailored for AI data centers. Here’s a detailed breakdown of its key capabilities:
- Comprehensive Simulation Capabilities: The tool provides detailed simulations of mechanical, thermal, networking, and electrical systems. This allows operators to understand the interdependencies between different systems and optimize their performance accordingly.
- Real-Time Data Integration: The digital twin is continuously updated with real-time data from the data center’s sensors, meters, and other monitoring devices. This ensures that the virtual replica accurately reflects the data center’s current state.
- “What-If” Scenario Analysis: The tool enables operators to run “what-if” scenarios to evaluate the impact of different changes or events on the data center’s performance. This helps them make informed decisions about capacity planning, maintenance, and incident response. For instance, operators can simulate the impact of adding new AI servers, adjusting cooling settings, or experiencing a partial power failure.
- Predictive Maintenance Features: The digital twin incorporates predictive maintenance algorithms that analyze historical data and real-time sensor readings to identify potential equipment failures. This allows operators to schedule maintenance proactively and prevent downtime.
- Chip-Level Modeling: A unique feature of this tool is its ability to conduct modeling on load behavior down to the chip level. This provides unprecedented visibility into the power consumption and thermal characteristics of individual AI processors, enabling operators to optimize cooling and power distribution at a granular level.
- Integration with Nvidia Omniverse: The tool leverages Nvidia’s Omniverse platform, a powerful platform for 3D design collaboration and simulation. This allows users to visualize the data center in a realistic 3D environment and collaborate on design changes in real-time.
Deep Dive into Simulation Capabilities
The simulation capabilities of the Schneider Electric-ETAP digital twin are extensive, covering a wide range of data center systems. In terms of mechanical systems, the tool can simulate the performance of cooling systems, including chillers, cooling towers, and computer room air conditioners (CRACs). It can also model the airflow and temperature distribution within the data center, allowing operators to identify hotspots and optimize cooling strategies. The simulation of thermal systems is crucial for maintaining optimal operating temperatures for IT equipment and preventing overheating. This includes simulating heat transfer, fluid dynamics, and thermodynamic processes within the data center environment. These simulations can help identify potential thermal bottlenecks and optimize cooling system design.
Networking simulations enable operators to model the performance of the data center’s network infrastructure, including switches, routers, and cabling. This allows them to identify potential bottlenecks and optimize network configuration for AI workloads. Electrical system simulations are equally critical, enabling operators to model the power distribution network, including transformers, switchgear, and uninterruptible power supplies (UPS). The tool can simulate power flow, voltage drop, and fault conditions, allowing operators to ensure the reliability and stability of the power supply. The ability to simulate the electrical system is particularly important for AI data centers, which often have high power densities and stringent power quality requirements.
Real-Time Data Integration and its Importance
The real-time data integration feature of the digital twin is essential for maintaining an accurate and up-to-date representation of the data center. The tool connects to a variety of sensors, meters, and monitoring devices within the data center, collecting data on temperature, humidity, power consumption, airflow, and other key parameters. This data is then used to update the digital twin in real-time, providing operators with a dynamic view of the data center’s current state. The real-time data integration capability allows operators to identify and respond to potential problems before they escalate. For example, if the digital twin detects a sudden increase in temperature in a particular area of the data center, operators can investigate the cause and take corrective action before equipment is damaged. Similarly, if the digital twin detects a power outage, operators can quickly identify the affected systems and implement backup power solutions.
The accuracy of the real-time data integration is paramount. The digital twin relies on the accuracy and reliability of the data it receives from sensors and other monitoring devices. Therefore, it is important to ensure that these devices are properly calibrated and maintained. Data validation and cleansing techniques should also be used to identify and correct any errors in the data. Furthermore, the digital twin should be able to handle missing or incomplete data gracefully. This can be achieved by using data imputation techniques to fill in the gaps in the data.
Expert Perspectives and Industry Implications
Industry experts recognize the potential of digital twins to revolutionize data center management, particularly in the context of AI.
According to Tanuj Khandelwal, CEO of ETAP, “We’re fundamentally reimagining how data centers can be designed, managed, and optimized in the AI era. By bridging electrical engineering with advanced virtualization and AI technologies, we’re creating a new paradigm for infrastructure management.”
Dion Harris, senior director of high-performance computing and AI factory solutions at Nvidia, emphasizes the visibility and control the tool provides: “We’re offering data center operators unprecedented visibility and control over power dynamics, empowering them to optimize their infrastructure and accelerate AI adoption while enhancing operational resilience.”
Pankaj Sharma, EVP for data centers, networks and services at Schneider Electric, highlights the importance of collaboration: “Collaboration, speed, and innovation are the driving forces behind the digital infrastructure transformation that’s required to accommodate AI workloads. Together, ETAP, Schneider Electric, and Nvidia are not just advancing data center technology — we’re empowering businesses to optimize operations and seamlessly navigate the power requirements of AI.”
The introduction of this digital twin tool represents a significant step forward in the evolution of data center management. It addresses the unique challenges posed by AI workloads and provides operators with the tools they need to optimize their infrastructure, improve efficiency, and enhance resilience.
Analysis of Expert Opinions
The expert opinions highlight several key themes related to the impact of digital twins on data center management. The first theme is the potential for digital twins to fundamentally reimagine how data centers are designed, managed, and optimized. This reflects the belief that digital twins can provide a more holistic and data-driven approach to data center management, enabling operators to make better decisions based on real-time insights. The second theme is the importance of collaboration and innovation in driving the digital infrastructure transformation that is required to accommodate AI workloads. This reflects the recognition that no single organization can solve all of the challenges associated with AI data centers. Collaboration between different companies with complementary expertise is essential. The third theme is the focus on empowering businesses to optimize operations and seamlessly navigate the power requirements of AI. This reflects the understanding that AI workloads have unique power and cooling requirements that must be addressed in order to ensure optimal performance and reliability.
Counterarguments and Alternative Perspectives
While the benefits of digital twins are compelling, it is important to consider potential challenges and alternative perspectives.
- Implementation Costs: Implementing a digital twin solution can be expensive, requiring significant investments in hardware, software, and personnel. Small and medium-sized data centers may find the cost prohibitive.
- Data Security Concerns: Digital twins rely on the collection and analysis of large amounts of data, which raises concerns about data security and privacy. Data center operators must implement robust security measures to protect sensitive information.
- Complexity: Creating and maintaining an accurate digital twin requires a deep understanding of data center operations and advanced modeling techniques. This can be challenging for organizations that lack the necessary expertise.
- Alternative Approaches: Some data center operators may prefer to rely on traditional monitoring and management tools, such as building management systems (BMS) and data center infrastructure management (DCIM) software. These tools can provide valuable insights into data center operations, although they may not offer the same level of detail and predictive capabilities as a digital twin.
Exploring Implementation Challenges in Detail
The implementation of a digital twin is not without its challenges. The initial investment in hardware, software, and training can be substantial. High-fidelity sensors, advanced simulation software, and skilled personnel are essential for building and maintaining an effective digital twin. Data integration can also be a significant challenge. Data centers often have a variety of systems and devices that generate data in different formats. Integrating this data into a single digital twin can be complex and time-consuming. Ensuring data quality is another important challenge. The accuracy and reliability of the digital twin depend on the quality of the data it receives. Data validation and cleansing techniques should be used to identify and correct any errors in the data. Furthermore, change management can be challenging. Data centers are constantly evolving as new equipment is added and workloads change. The digital twin must be updated to reflect these changes, which requires ongoing effort and expertise.
Scalability is an additional factor to consider. The digital twin should be able to scale to accommodate the growing size and complexity of the data center. This may require the use of cloud-based infrastructure and distributed computing techniques. Finally, organizational culture can be a barrier to adoption. Some organizations may be resistant to change and may be reluctant to invest in new technologies. It is important to build a strong business case for the digital twin and to communicate the benefits to all stakeholders.
Conclusion
The Schneider Electric-ETAP digital twin tool represents a significant advancement in data center management, particularly for facilities supporting AI workloads. By providing a comprehensive virtual representation of the data center, the tool enables operators to optimize their infrastructure, improve efficiency, and enhance resilience. While there are challenges associated with implementation and data security, the potential benefits of digital twins are undeniable. As AI continues to transform industries and drive the demand for specialized data centers, digital twins are likely to become an increasingly important tool for managing and optimizing these critical facilities. The collaboration between Schneider Electric, ETAP, and Nvidia showcases the power of combining expertise from different domains to address the complex challenges of the AI era. This innovation promises to empower businesses to seamlessly navigate the power and operational demands of artificial intelligence, marking a new era in data center technology and infrastructure management. The future of AI data centers is being shaped by these kinds of advanced, collaborative solutions, driving innovation and efficiency in the digital landscape.
Word count: 1737 “`